Currently Empty: $0.00
Blog
What is Deep Learning?
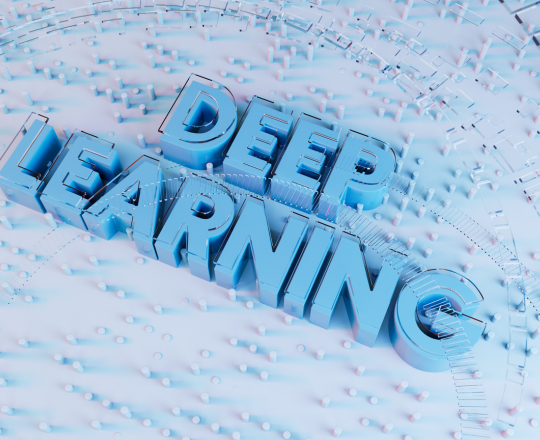
In the rapidly evolving field of artificial intelligence (AI), Deep Learning has emerged as a groundbreaking technology driving transformative advancements across industries. Deep Learning is a specialized branch of machine learning that uses artificial neural networks to mimic the way humans learn and process information. It is designed to recognize patterns, make predictions, and solve complex problems by analyzing large datasets. But what exactly is Deep Learning, and why is it so impactful? Let’s dive deeper.
Understanding Deep Learning
At its core, Deep Learning involves training computers to learn from data using artificial neural networks. These networks are composed of multiple layers of interconnected nodes (neurons), which process and transform data step by step. This multi-layered approach allows the system to learn features hierarchically, starting with basic elements and building up to more complex representations.
The term “deep” refers to the depth of these layers in the neural network. Traditional machine learning models might involve one or two layers, but deep learning networks can include dozens or even hundreds of layers, enabling them to tackle highly intricate tasks.
How Does Deep Learning Work?
Deep Learning models function through three primary components:
- Input Layer: The data enters the model here, such as images, text, or numerical values.
- Hidden Layers: These intermediate layers perform computations to extract features and identify patterns. Each layer’s output serves as the input for the next layer.
- Output Layer: The final layer produces the desired outcome, such as a classification, prediction, or decision.
Each neuron in a layer is connected to neurons in adjacent layers, with each connection having a weight. These weights are adjusted during the training process using a technique called backpropagation, which minimizes the difference between the model’s predictions and actual results.
Applications of Deep Learning
Deep Learning has reshaped industries with its ability to achieve human-like performance in various domains. Here are some notable applications:
Computer Vision:
- Facial recognition systems (used in smartphones and security systems).
- Self-driving cars that detect objects, pedestrians, and road signs.
- Image editing tools powered by AI.
Natural Language Processing (NLP):
- Language translation services, such as Google Translate.
- Sentiment analysis to understand customer feedback.
- Chatbots and virtual assistants like Siri and Alexa.
Healthcare:
- Diagnosing diseases from medical images such as X-rays or MRIs.
- Drug discovery through pattern analysis in molecular data.
- Personalized treatment recommendations.
Finance:
- Fraud detection systems.
- Stock price predictions.
- Risk assessment for loans and insurance.
Entertainment:
- Content recommendations on platforms like Netflix and Spotify.
- AI-generated art and music.
Why is Deep Learning Important?
- High Accuracy: Deep Learning models often outperform traditional machine learning techniques, especially in tasks like image recognition and speech processing.
- Scalability: With the availability of large datasets and powerful computational resources, Deep Learning models can handle vast amounts of information effectively.
- Automated Feature Engineering: Unlike traditional machine learning, which requires manual feature selection, Deep Learning automatically extracts the most relevant features from raw data.
- Wide Range of Applications: From diagnosing diseases to powering autonomous vehicles, Deep Learning has shown unparalleled versatility.
Challenges in Deep Learning
While Deep Learning is powerful, it comes with certain challenges:
- Data Dependency: Deep Learning models require vast amounts of labeled data for training, which may not always be available.
- Computational Power: Training deep neural networks demands significant computational resources, such as GPUs and TPUs.
- Interpretability: The complexity of these models makes it difficult to understand how they arrive at specific decisions.
- Overfitting: Without proper regularization, models may perform well on training data but fail to generalize to new, unseen data.
Conclusion
Deep Learning has revolutionized the field of AI, enabling breakthroughs that were once thought impossible. Its ability to process and analyze massive amounts of data with high accuracy makes it a cornerstone of modern technology. Whether it’s driving self-driving cars, diagnosing diseases, or powering virtual assistants, Deep Learning continues to shape the future of innovation.
As industries increasingly adopt Deep learning solutions, understanding its fundamentals and applications becomes crucial for anyone aspiring to thrive in the data-driven world. Are you ready to explore the limitless potential of deep Learning?